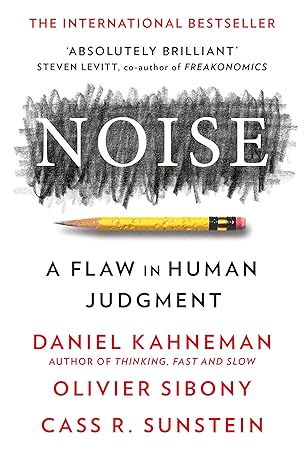
Noise
System noise is unwanted
Noise can’t be avoided, but it can be reduced. In noisy systems, errors do not cancel each other out; they add up. An overpriced and an underpriced error may on average, look right, but the company has made two costly errors. Unwanted variability in judgements can cause serious problems including loss of money and rampant unfairness.
Informal and partly subjective judgements are not always entirely consistent; diversity of taste can help account for errors, and some noise is inevitable.
You don’t need to know the target or the bias to recognise and measure noise.
We learn to agree with our past selves.
Increasing fluency and ease of the subjective experience of decision-making has us believing that we have got this. Newbies discuss while experts believe. We become confident in our judgement simply by exercising our judgement. Harmony and consensus are preferred over dissent and conflict.
Wherever there is judgement, there is noise and more of it than we think. A noise audit shatters the illusion of agreement.
Unwanted variance is easy to define and measure when interchangeable professionals make decisions. Personal experiences that make you who you are are not truly relevant to this decision. Instead, apply probabilistic thinking.
A matter of taste
We seek the achievement of a coherent solution; an internal signal of judgement completion. A sense of coherence is part of the judgement experience when the focus should be on making the best judgement.
Examine the quality of the process that led to the decision.
Evaluative judgements
A sentence handed down in court is an example of an evaluative judgement. Evaluative judgements depend partly on the values and tastes of those making them.
We can predict how things will perform, but when information comes in, the final decision is an evaluative one.
Don’t mix your values and your facts
Good judgements are based on objective facts, not fears and hopes.
The decision on when to leave for the bus station should be based first on the probability of travel time. Consideration of costs such as wasted time should follow the assessment of facts.
Measurement errors add up they don’t cancel out. On average it is not helpful.
A reduction in noise is equally impactful as a reduction of bias on overall error.
Maximum precision (the least noise).
Maximum accuracy (the least bias).
The standard deviation (SD) from the mean estimates the level of system noise. Calculating SD doesn’t require knowledge of the true value. To calculate bias you need the true value.
The error equation does now work for evaluative judgements. One minute late is the same as five minutes late for a bus; errors are asymmetrical.
System noise
System noise refers to the undesirable variability in the judgment of the same case by multiple individuals.
Level noise is the variability in the average level of judgement by different judges.
Pattern noise refers to the variability in judges’ responses to particular cases, think inter and intra-rater variability.
Occasion noise
Criminal sentences often depend on the mood of the judge or the temperature outside. You are not the same person at all times. Think basketball player taking free throws.
Two wrongs don’t make a right but it may influence your decision. For example, if you said “no” twice you may say “yes” next just to try balancing things out.
You are not always the same person, and you are less consistent over time than you think but you are more similar to yourself yesterday than you are to another person today.
Occasion noise is not the largest source of system noise.
The impact of mood on judgement is worth considering.
Groupthink
Wisdom in crowds relies on independence of thought.
Early popularity is key. Popularity is self-reinforcing. The best and the worst typically find their place but the rest is not up for debate but social influence and this creates noise.
Who speaks first influences group dynamics.
Averaging the independent judgements of different people generally improves accuracy.
Get a second opinion, alternatively, if a second opinion is not an option, actively argue against yourself to find another perspective. Seek an outside view where possible. And always average the estimates, think Francis Galton’s Ox.
Predictive Judgements
In predictive judgements, humans weigh predictors differently and inconsistently compared to a computer. For example, we might value motivation over technical skills, something a computer won’t do unless instructed.
Mechanical judgements made using simple models outperform clinical judgements. While we might believe our thinking is subtler, more insightful and more nuanced we are mostly noisier. For example, no amount of motivation will overcome a severe skill deficit and vice versa. If we weigh motivation over skill we are going to increase noise.
Complex rules are rarely true, and even if they are, they apply in conditions that are rarely observed. Subtly, complexity and richness don’t help human judgement.
Increasing the complexity of the inputs will enhance predictive accuracy.
Simple models of judgment help eliminate subtleties and pattern noise. While some subtleties are valid, many are not. Complex rules often give us a sense of illusion of validity.
Objective Ignorance
We can expect people engaged in predictive tasks to underestimate their objective ignorance, which consists of intractable uncertainty (what cannot possibly be known) and imperfect (what could be known but isn’t). We need more information and less objective ignorance.
Experts can tell a great story about how things are going to play out and why but they lack the ability to accurately predict the results. Denial of ignorance. Rarely is this level of confidence justified. Characteristics include:
Predictably of events that are in fact unpredictable.
Denial of the presence of bias and noise.
Relying on gut feeling.
Focusing on causation rather than correlation. Knowing without knowing why.
Practice conscious ignorance instead, it can expand your perspective and open up infinite possibilities.
Correlation does not imply causation
A common misconception is that events that couldn’t have been predicted can nevertheless be understood. To claim to understand is to describe a causal chain. The ability to make an accurate prediction serves as a measure of whether such a causal chain has indeed been identified.
If we can’t predict events with accuracy we don’t understand those events.
Correlation, the measure of predictive accuracy, is a measure of how much causation we can explain. The reverse is not true – causation does imply correlation.
For instance, a child’s shoe size increases as does a child’s knowledge of math. However, a bigger shoe size does not cause you to be better at math. If you know a child’s shoe size you could predict a math ability but not the cause.
Once an end point is known causal thinking makes it feel entirely explainable, indeed predictable. Events appear normal in hindsight although they weren’t expected and we could not predict them. Our understanding of reality is backwards facing, we retrofit, and we make it fit a story that we can understand.
Substitution and Confirmation bias
Rather than answer a difficult question we find an answer we like to an easier question.
The question of “How probable?” differs from “How similar?” We substitute probability for similarity and make the question easier. Probability is constrained by a certain logic.
We create psychological biases; and predictable errors.
Take the outside view; rather than an isolated case, think of it as a member of a class of similar cases. Think statistically about the class, instead of thinking casually about the focal case.
Substitution bias; Misweighting of the evidence.
Conclusion biases; bypassing or considering in a distorted manner. Our judgements are easily prompted, we jump to conclusions and then stick to them.
Excessive coherence; Amplifying the effect of initial impressions and reducing the impact of contradictory information.
Base rate neglect; Focusing on the availability of information rather than assessing frequency; availability heuristic.
Substitution examples: Do I believe in climate change vs. do I trust the people who say it exists? We fall for a nice-looking CV.
Confirmation bias: I don’t like it and I don’t believe it, we confirm our thinking.
People determine what they think by consulting their feelings.
Psychological biases can contribute to statistical bias if many people share the same biases. However, in many cases, where individuals vary in their biases these psychological biases create system noise.
Illusion of agreement
If people cannot imagine possible alternatives to their conclusions they will naturally assume that other observers must have reached the same conclusion.
We are not always confident about our decisions but most of the time we are more confident than we should be.
When the triggers of pattern noise are rooted, in our personal experiences and values, we can expect the pattern to be stable, a reflection of our uniqueness.
Pattern noise: stable pattern noise + occasion noise. Occasion noise ensures we are idiosyncratic.
Respect experts
No objective way to evaluate their judgements. If we can’t authenticate results we should probably avoid thinking they are an expert.
Respect experts; they are excellent at creating coherent stories. Facts are easily fitted into a coherent story that inspires confidence. Experience enables the recognition of patterns, and hypotheses are formed and confirmed quickly.
Rethinking leadership. Being actively open-minded is helpful in making good judgements. If we think of leaders as being decisive, it is better that they are decisive at the end of the process, not the start.
Bias is directional
Think about modifying the environment in which a decision is made, ex-ante. The aim is to reduce biases or enlist biases to make a better decision, for example, automatic enrolment into pensions.
The issue, of course, is that it’s difficult to know which biases are present.
For example, the planning fallacy can be addressed by adding in a 30% contingency. That works if the bias is not already built in. These approaches work where the general direction of error is known and manifests itself as a clear statistical error. However, we are rarely aware of biases if we are being misled by them.
We recognise biases in others more easily than we do in ourselves.
Checklists have a long history of improving decisions in high-stakes contexts and are particularly well suited to preventing the repetition of past mistakes.
Bias is an error we can often see and even explain. It is directional. This is why an observer can hope to diagnose biases in real time as a decision is being made.
Think of noise reduction as personal hygiene. We cannot easily see or explain. It is an unpredictable error.
Noise prevention will prevent many errors; you just won’t know which ones.
Asymmetrical bias
ACE V is an acronym used by fingerprint experts.
Analyse: Assess whether the fingerprint is of a sufficient quality for comparison.
Comparison: Compare it to an exemplar print.
Evaluation: Reach a conclusion of Inconclusive, Identification or Exclusion.
Verification: Have another examiner verify the findings.
The influence of biasing information can alter our perception and how we interpret it.
Confirmation bias is a problem. When an examiner knows it’s a positive identification, it can further confirmation bias, leading to the replication and amplificationof errors creating a confirmation cascade.
Due to the trust placed in fingerprint identification by the judicial system, the cost of error is asymmetrical. A false positive is a deadly sin and fingerprint experts are trained to be cautious. Therefore, bias is not the same in both directions. It is less risky to bias toward inconclusive than false positives.
Reducing Noise
The first step to reducing noise is to acknowledge its possibility.
Three ways to reduce noise:
- Training. Averaging predictions. Noise bias audit and considering reference classes.
- Teaming (a form of aggregation) open book management. See and debate predictions to encourage open-mindedness and opposing arguments.
- Selection. Super predictors into elite teams.
Be exposed to information only as you need it. Sequence information.
Documenting judgements at each stage.
Revisit your decision repeatedly to mitigate against occasion noise.
Blind the decision makers to avoid influence.
Aim to keep a shadow of doubt.
Improving forecasting
The average of a noisy group will be more accurate than the average of a unanimous one. Average the forecasts.
Use the best forecasters. Pick the best predictor and others who offer a different perspective rather than similar predictors who are only less accurate than the best predictor.
Use the Delphi method or “Estimate-Talk-Estimate.
Eliciting and aggregating a diverse opinion that is derived independently is the quickest and easiest decision hygiene strategy.
Strive to be in perpetual beta.
Favour relative judgements and relative scales.
Our ability to categorise objects on a scale is limited.
Use anchoring to provide examples and illustrate what a ranking score would look like.
Improving judgements by clarifying the rating scale and training people to use it consistently.
The Apgar score is a quick way for health professionals to evaluate the health of all newborns at one and five minutes after birth. Apgar score provides guidelines which produce very little noise. Agreement is high.
The Apgar score works because a complex decision is broken down into a number of easier smaller judgements on predefined dimensions.
Key elements:
Providing a focus on the relevant predictors.
Simplification of the predictive model.
Mechanical aggregation.
Consider a strategic decision as a series of smaller decisions.
Independently assessed each decision as part of a checklist.
Each assessment is an intermediate goal. All available information is assessed and one aspect of the decision does not weigh other choices that are unrelated. One fact. One assessment.
“Leaving aside the weight we should give this topic in the final decision, how strongly does the evidence on this assessment argue for or against the deal?”
Avoid seeking to take one big final decision as a way of wrapping up and moving on.
Rules and standards
Rules reduce the role of judgement. Standards delegate power. The work is to specify the meaning of open-ended terms.
Getting people to agree on noise reduction strategies is one reason standards not rules are in place. Lack of information is another reason. Often we use a mixture of rules and standards. Regardless, of rules or standards the focus should be on noise, bias or both.
Standards often produce a great deal of noise. Rules will largely reduce noise. Noise is often a product of a failure to issue rules.
When noise is loud and people can see that people are not being treated fairly there is often a movement toward rules. Often when the horse has bolted.
People don’t like being told what to do. The failure to follow rules also creates noise, if rules are deemed unfair or harsh, people will not follow them. Guidelines stifle discretion but reduce in noise.
For repeated decisions, rules might be the way to go.
When considering whether a rule or a standard is appropriate. First, ask which produces more mistakes. Then ask which is easier or more burdensome to produce or work with.
The cost of a decision is typically higher for standards compared to rules. When it is costly to decide what to do, discretion is preferred. Discretion is closely related to trust.
A teacher uses their judgement to score an essay but not a multiple choice exam.
Some judgements are predictable and some predictive judgements are verifiable but many are unverifiable and the only way to assess judgement is to examine the quality of the thought process.
Noise vs Bias
The absence of statistical thinking is one reason noise gets less attention than bias. Bias appeals to our need for a causal story. From an organisational perspective noise is an embarrassment.
Many judgements are evaluations and it is difficult to compare to a true objective value.
Six principles:
- The goal of judgement is accuracy not individual expression; rules and guidelines help
- Think statistically and take the outside view. People cannot be faulted for failing to predict the unpredictable, but they can be blamed for a lack of predictive humility.
- Structure judgements into several independent tasks. Avoid excessive coherence
- Resist premature intuitions. Intuition should be informed, disciplined and delayed. Sequence information; the man that knew too much.
- Obtain independent judgements from multiple judges then aggregate those judgements. Independence is critical to this principle. Collect judgement prior to any discussion.
- Favour relative judgements and relative scales. Our ability to categorise objects on a scale is limited.
Is it worth it?
The cost-benefit of reducing noise is a key factor in the need to reduce noise. Figure out how much noise is present and its cost. Then decide if it’s worth reducing noise.